Leading the Charge in Tech & Thought.
Stay ahead of the curve with our blogs. Our experts break down topics from Generative AI to cutting-edge Data Engineering, offering insights and tips for businesses eager to grow. Whether you're looking to stay informed or get inspired, our blogs offer a wealth of resources on the latest tech trends.
WHAT WE DO.
Explore our wide gamut of digital transformation capabilities and our work across industries.
Explore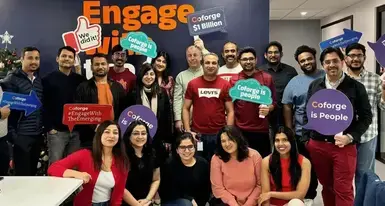