Introduction
Unstructured Data
In 1998, Merrill Lynch stated that most data stored in an enterprise is unstructured and estimated to be as high as 80%. This number may have been a bit anecdotal at the time with only a few parties accepting this number unequivocally. Though this number remained unverified, some sources suggested that the actual number may indeed be close to 80%.
Fast forward to 2020. IDC and Dell EMC predicted that by this year, there will be an increase of 40 zettabytes of data. Furthermore, IDC and Seagate reported that by 2025, the global datasphere will grow to 163 zettabytes and most of this data will be unstructured.
What do we observe from the above metric? Before making the deduction, we need to elucidate what ‘unstructured data’ means in the context of an enterprise. Unstructured data does not have a predefined structure and is usually written and presented in a free-flowing manner. The data could potentially include documents such as employee information, insurance policies, travel papers, legal contracts, agreements, invoices etc.
Making sense of this information stack to bring out themes and trends requires time and a huge effort on the part of the organization. As most of this data comes in as text, the language is ambiguous, and key messages buried in text data are not easy to discern or process. Also, as the merit remains in combining text data with structured data in decision-making contexts, the analysis of unstructured data remains a challenge.
Characteristics of Unstructured Data
Unstructured data does not conform to a data model Different forms include text (highest percentage), videos, images etc. This data does not follow a semantic or rule and cannot be stored as rows and columns in a database Unstructured data lacks a predefined format or sequence and has no discernible structure – it cannot be used by a computer program easily
Challenges of Document Processing
The numbers stated in the first paragraph clearly suggests that there is a massive influx of information coming into an organization. But there is a huge gap in visibility between this cohort of incoming data and an enterprise’s capacity to glean useful insights from said data. Unstructured data and excessive documentation has troubled organizations at many levels leaving a lot of potential business value untouched from an analytical standpoint.
What do we need to handle documents on a large scale?
Artificial Intelligence has yielded new solutions that focus on processing large vats of content documents. There is still a big gap between enterprise legacy systems and these new AI-based data solutions. But there is an upside. New advances in machine learning and data science have narrowed the gap significantly to eliminate manual intervention.
So how do we solve this document processing conundrum?
Document AI
Coforge has been working with leading global brands in transforming them at the intersect of unparalleled domain expertise and emerging technologies to achieve real-world business impact. In the world of document analytics, Coforge has developed Document AI – a business solution that leverages artificial intelligence to turn unstructured data into actionable information.
Document AI is an Intelligent Document Processing Accelerator
Features of Document AI
How Does Document AI Work?
The key feature of Document AI is that it provides Smart Document Processing in four simple stages
Stage 1: Extract Information
When one thinks of extracting data from a document, what examples come to mind? For us, one of the challenges was retyping tables / fund history from a prospectus which proved to be cumbersome. Another example was invoice processing which looks to be simple and structured. In the real world however, every invoice is different. Current template matching techniques fail to extract data effectively. Keeping this in mind, we ensured that our Smart Extraction for accessing any kind of data in any format / template was ready to go from day one. Once configured for what needs to be extracted, we could quickly extract data from templates and multiple tables, map their columns, and send them to a client’s structured data warehouse.
Stage 2: Provide Analytics for Extracted Content
In most cases, extraction is not the final solution. Rather, businesses today want to derive sensible insights from data / extracted text. Document AI provides features like clustering, classification, context understanding, summarizers, knowledge graph, sentiment analysis etc. to make the text data generate insights for businesses to leverage. All these options have been provided as a simple API call.
Stage 3: Attended and Unattended Options
How comfortable are clients with leveraging AI and Machine Learning? The answers often vary. Coforge offers two compelling options:
Attended: users are always scrutinizing the document, but the key fields are pre-mapped and highlighted in the document. This provides the time savings of AI coupled with the quality assurance of human verification
There is a façade of unstructured data that is often ignored – generation of useful content. Yes, you can also create it. Whether it is a fund report, regulatory filing, or a purchase order, our Smart Document Processing focuses on this tenet – content generation.
Stage 4: Integrate and Complete Publishing Based on Document Data
With our Document AI offering, one can quickly convert structured data into publishable structured data as per the requirement. This can be fed into downstream systems such as Appian etc. to further complete a process. An easy-to-use online editor and collaboration tool lets the user quickly transform data into reusable charts and graphs and further, provide a place to collaborate on a people-driven context. As an example, we can combine Document AI with Appian for one-click regulatory filings.
Let us now look at a couple of business cases on how Document AI addressed challenges of unstructured data points.
Business Cases Document AI: Agreement Processing
Document AI: Empower MSLs by Enriching Data
Medical Science Liaison (MSL) plays a vital role in pharmaceutical and healthcare industries
Business Case
To ensure that products are utilized effectively by scientific experts and internal colleagues Establish and maintain a peer-to-peer relationship with leading physicians Enrich congress data and get insights to enable MSL Solution Develop capabilities to read congress abstracts Expose focus areas and extract usable inferences from the abstracts Develop a standard taxonomy / hierarchy Feed insights to customers’ in-house platforms Document AI platforms are then used for solving the above roadblocks
Document AI: Ownership Graph of Companies
Business Case
Tax reduction through shifting of profits from high tax countries by using debt shifting, registering intangible assets, and strategic transfers pricing The auditors were faced with the challenges of high volume, multi-level ownership, and circular trading transactions
Solution
Conclusion
Unstructured data is a huge challenge. For large organizations, the issue amplifies further. The challenge with the organization is not just limited to organizing and storing data but also gaining insights from it. AI solutions developed by an able technology partner can easily enable organizations to bring order to their data while also enabling them to gain valuable insights from that data.
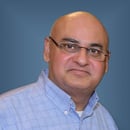
Vic is the Executive Vice President, Microsoft Business Unit at Coforge. He is responsible for spearheading the growth of the Cloud service line across geographies. With over 2 decades of experience in the industry and having worked in leadership positions in companies like Microsoft, Vic has immense domain expertise in emerging technologies like Cloud and is driving business transformation, innovation and sustainability.
Related reads.
About Coforge.
We are a global digital services and solutions provider, who leverage emerging technologies and deep domain expertise to deliver real-world business impact for our clients. A focus on very select industries, a detailed understanding of the underlying processes of those industries, and partnerships with leading platforms provide us with a distinct perspective. We lead with our product engineering approach and leverage Cloud, Data, Integration, and Automation technologies to transform client businesses into intelligent, high-growth enterprises. Our proprietary platforms power critical business processes across our core verticals. We are located in 23 countries with 30 delivery centers across nine countries.