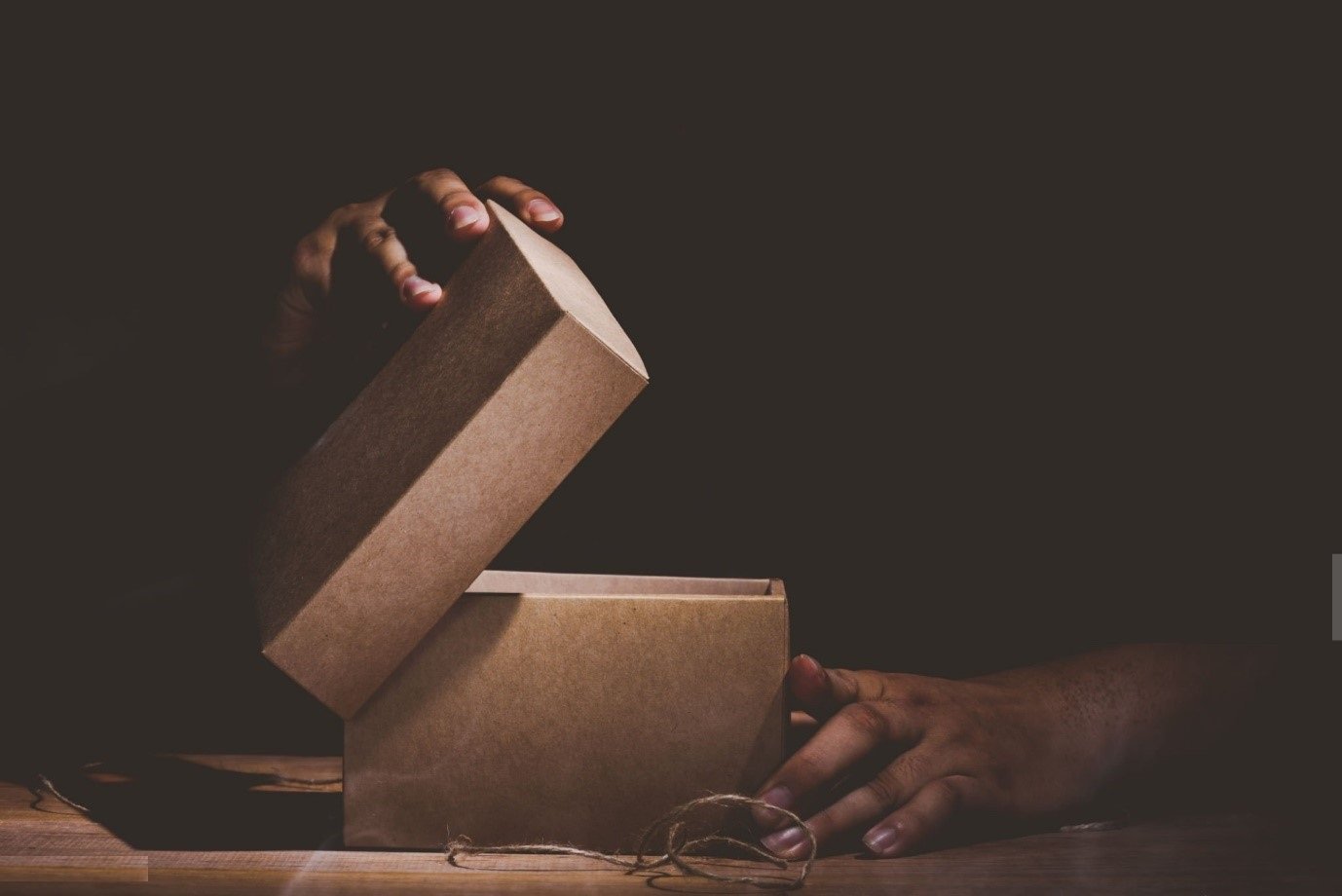
As we look ahead at 2023, it is clear that AI is playing a huge role in our daily lives, and we are looking at solving global issues whether it is diagnostic testing for terminal diseases or processing insurance claims or getting a loan approved. With these strides in technology, the role of Responsible AI has become a talking point. It’s an approach to developing and deploying AI in an ethical manner so as to make the process more transparent and fair.
Problems in deployment of AI technologies
AI applications use Machine learning models that are complex and often use sophisticated algorithms. It can be difficult for humans to understand how these make their predictions or decisions. This can make it challenging for insurers, for example, to explain their decisions to customers or regulators and can also make it difficult to identify and correct any biases or errors in the models.
This black box approach of AI can lead to a lack of trust in the decisions and predictions made by the AI model. When the inner workings of an AI model are not transparent or easily understood, it can be difficult for people to have confidence in the accuracy and fairness of the model's outputs. This can be particularly problematic in industries where decisions made by AI have significant consequences, such as in finance and healthcare where the decisions made can impact society.
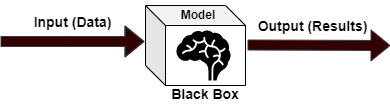
There are a number of problems associated with the development and deployment of AI technologies. Some of the problems include:
- Bias and discrimination - AI systems are often trained on biased data, leading to perpetuation of biases and discrimination in decision-making processes.
- Lack of transparency - AI systems are often opaque, making it difficult to understand how decisions were made and leading to concerns about accountability.
- Privacy and security - AI systems often collect and process personal data, raising concerns about privacy and security.
- Unintended consequences - AI systems can have unintended consequences, such as automating harmful processes or perpetuating existing inequalities.
- Lack of human control - AI systems could operate autonomously, raising concerns about human control and accountability.
These problems can lead to negative impact on individuals and society, erode trust in the technology, and hinder its adoption and potential benefits. The emergence of responsible AI has helped to address these and other issues, by promoting ethical, transparent, and trustworthy development and deployment of AI technologies. By addressing these problems, responsible AI is helping ensure that AI is used for the benefit of society, while minimizing its negative impacts.
Overview
Responsible AI refers to the development and deployment of AI technologies in an ethical and accountable manner, considering the potential impact on individuals, society, and the environment. It’s an emerging area of AI governance and the word ‘Responsible’ covers both ethical and legal aspects of AI usage. This includes considering issues such as fairness, transparency, privacy, security, accuracy, accountability, explainable, and human control over AI systems and taking steps to mitigate harmful outcomes. The goal of responsible AI is to ensure that AI is used for the benefit of humanity, rather than causing harm or perpetuating the existing inequalities.
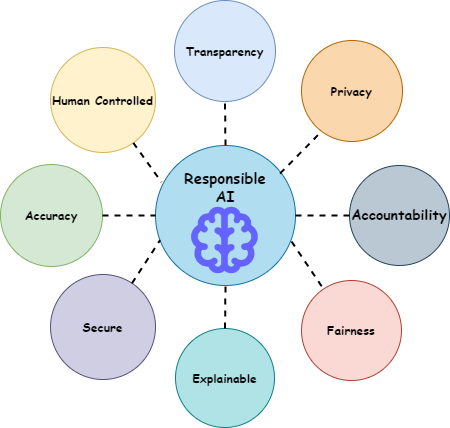
One key aspect of Responsible AI is transparency. This means that the decision-making process and outcomes of AI systems should be explainable and transparent to developers, regulators and stakeholders. This allows for greater accountability and trust in the AI systems and helps identify and mitigate any potential biases and errors. Transparency can be promoted through the following actions:
- Providing information about how AI systems work, what data they use, and how they make decisions.
- Make the source code of AI system available for review and analysis.
- Providing clear and concise explanations of how the decisions are made by AI systems.
- Documenting the data inputs and processing steps used by AI systems to generate outputs.
- Facilitating access to relevant documentation and training materials, so that users can better understand the technology.
Another important aspect of Responsible AI is Fairness. AI systems should be designed and trained to be fair, unbiased, and inclusive, avoiding discrimination based on any protected attribute and ensuring equal opportunities for all individuals. This is particularly important in applications such as hiring, lending and criminal justice, where AI systems have the potential to perpetuate and even amplify existing societal biases. Fairness can be ensured in AI systems through the following actions:
- Collecting diverse and representative data for training AI systems, to reduce the risk of bias and determination.
- Providing explanations and justifications for decisions made by AI systems, to promote transparency and accountability.
- Regularly testing and auditing AI systems to identify and address any biases or discrimination that may be present.
- Ensuring that the design of AI systems takes into account the needs of diverse groups of people, and that technology is accessible and usable by all.
- Engaging with diverse groups of stakeholders, including those who may be impacted by AI systems, in the development and deployment of technology.
Accountability is also a crucial aspect of Responsible AI. It means that those who develop, deploy, and operate AI systems are responsible for their outcomes, including any negative impacts. This includes not only technical accountability, but also legal and ethical accountability, as well as Transparency and Explainability of the decision-making process. Accountability can be promoted in AI systems through the following actions:
- Designing AI systems to be transparent and explainable, so that their decision-making processes can be understood and evaluated.
- Establishing clear lines of responsibility and accountability for AI systems, including identifying who is responsible for their development, deployment and maintenance.
- Conducting regular audits and evaluations of AI systems to identify any issues or negative impacts and taking corrective action when necessary.
- Establishing clear processes for handling complaints and grievances related to AI systems and ensuring that these processes are accessible and transparent.
- Ensuring that there are legal and regulatory frameworks in place to govern the development, deployment and usage of AI systems, and that those who violate these frameworks are held accountable.
Explainability is a critical component of Responsible AI. Explainable AI (XAI) refers to the development and use of artificial intelligence (AI) systems that can provide an explanation of how they arrived at a particular decision or prediction. This will help ensure that AI is used in an ethical and accountable manner, and that negative impacts are minimized. Explainability also helps to build trust in AI and increases the public confidence in its use. Explainability can be promoted in the following ways:
- Ensuring that AI systems can provide clear and concise explanations of how decisions are made, including what data was used and what factors were considered.
- Providing users with the ability to query AI systems and obtain explanations for specific decisions and outcomes.
- Documenting the data inputs and processing steps used by AI systems to generate outputs, to facilitate understanding and evaluations.
- Facilitating access to relevant documentation and training materials, so that users can better understand the technology and its decision-making processes.
Privacy is an important aspect of Responsible AI, as AI systems often collect, process, and analyse large amounts of personal data. In order to ensure privacy in the development and deployment of AI systems, following principles should be considered:
- Data protection – storing in a secure, privacy-friendly manner in accordance with relevant data protection regulations.
- Data minimization – Collecting only minimum amount of personal data necessary to achieve the intended purpose of the AI system.
- Fair and transparent data use – Ensuring personal data is used in fair and transparent manner.
- User control – Giving individuals control over their personal data -> access, modify and delete.
- Data security – prevent unauthorized access, modification, or misuse.
Accuracy refers to the degree to which an AI system produces correct results. In the context of Responsible AI, accuracy is an important consideration because errors and inaccuracies in AI systems can lead to harmful outcomes and erode public trust in the technology. Following steps can be taken to promote accuracy:
- Data quality – Ensuring that the data used to train AI systems is of high quality, diverse, and representative of the populations and situations in which the AI system will be used.
- Model evaluation – Regularly evaluating and testing AI systems to ensure they are functioning as intended and producing accurate results. In more desirable scenarios, it would be beneficial to establish a continuous model evaluation pipeline, that ensures the accuracy of model is not impacted once deployed because of data and concept drift.
- Error analysis – Conducting the root cause analysis of errors and inaccuracies, to identify and address the underlying causes of these issues.
- Continuous improvements – Continuously improving AI systems based on feedback, changes in the underlying data, problem statement, and any new developments in the field, with a focus on maximizing accuracy and reducing errors.
By promoting accuracy in AI systems, organizations can help to ensure that AI is used in a reliable and trustworthy manner, and that negative impacts are minimized.
Response AI is human-centred, which means that it considers the needs, values and well-being of individuals and society. It prioritizes human autonomy and ensures that the development and use of AI aligns with the values and goals of the society.
Human-centred responsible AI recognizes that AI technology can have far-reaching social, economic, and ethical implications, and seeks to involve a diverse range of stakeholders in the design and development process. This includes engaging with end-users, domain experts, decision makers, policymakers, and community organizations to ensure that AI systems are aligned with the needs and interests of the people they are designed to serve.
The following points should be considered to ensure that the AI systems are developed, deployed and used in a human-centred way
- User-centred design: AI systems should be designed with the end-users in mind, and their needs, values, and experiences should be considered throughout the design and development process. This can be achieved through techniques such as user research, prototyping, and usability testing.
- Transparency: AI systems should be transparent about how they make decisions and what data they use to make these decisions. This can help build trust between users and the technology and enable users to make informed decisions about their interactions with the system.
- Inclusivity: AI systems should be designed to be inclusive of all users, regardless of their race, gender, ethnicity, or other protected attributes. This can be achieved through techniques such as diverse data sets, bias mitigation strategies, and accessibility features.
- Ethics and human rights: AI systems should be designed to respect fundamental human rights, including privacy, security, and freedom from discrimination. Ethical considerations should be integrated into the design and development process from the beginning.
- Governance: AI systems should be governed by transparent, accountable, and participatory processes that involve a diverse range of stakeholders, including end-users, domain experts, and community organizations.
Concluding notes
In conclusion, responsible AI is not only a moral imperative but also a strategic priority for organizations and society as a whole. As AI continues to transform every aspect of our lives, it is crucial to ensure that it is developed and used in a way that promotes human values, rights, and well-being. By adopting responsible AI principles and practices, we can minimize the potential harms and maximize the potential benefits of AI, from enhancing healthcare and education to advancing sustainability and innovation. However, achieving responsible AI requires a collective effort and ongoing dialogue among researchers, developers, policymakers, civil society, and the public to foster a shared understanding and vision of the role and impact of AI in shaping our future.
About Coforge
Coforge is a global digital services and solutions provider, that enables its clients to transform at the intersect of domain expertise and emerging technologies to achieve real-world business impact.
We can help refine your problem statement, crystallize the benefits, and provide concrete solutions to your problems in a collaborative model.
We would love to hear your thoughts and use cases. Please reach out to Digital Engineering Team to begin a discussion.
Authors
Deepak Saini
Deepak Saini is AVP, Digital Services, Coforge Technologies. He has 23 years of IT experience with strong technology leadership experience in Machine Learning, Deep Learning, Generative AI, NLP, Speech, Conversational AI, Contact Center AI, Responsible AI.
Read more blogs
Siddharth Vidhani
Siddharth Vidhani is an Enterprise Architect in Digital Engineering of Coforge Technologies. He has more than 18 years of experience working in fortune 500 product companies related to Insurance, Travel, Finance and Technology. He has a strong technical leadership experience in the field of software development, IoT, and cloud architecture (AWS). He is also interested in the field of Artificial Intelligence (Machine Learning, Deep Learning - NLP, Image recognition, XAI).