We have entered an era fueled by AI, thanks to the declining costs of data storage and processing, improved accessibility and connectivity for everyone, and swift advancements in AI technologies. Machines powered by Artificial Intelligence are customizing recommendations for digital content based on individual tastes and preferences. They are creating clothing lines for retailers and, notably, are starting to outperform experienced doctors in identifying indications of cancer.
Banking stands out as one of the three primary sectors poised to experience the most significant impact from artificial intelligence. A report from McKinsey suggests that the implementation of AI technologies has the potential to provide an additional value of up to $1trillion annually.
In this article we will explore meaningful perspectives on the importance of AI in the banking sector, utilizing Coforge’s examples and case studies. Additionally, we will examine the challenges and constraints faced during the integration of AI into banking processes. We will then outline a strategic path forward, providing a preview of upcoming trends in this dynamic landscape.
AI and Generative AI have the potential to deliver significant new value to banks. Banks across the board will likely see an economic boost, with the biggest winners being the corporate and retail sectors. While the initial focus of Generative AI pilots in banking has been on boosting productivity in response to economic pressures, the technology holds significant transformative potential. It could fundamentally alter how tasks are performed, customer engagement occurs, and even pave the way for entirely new business models within the financial sector.
The Dominance of AI applications in the banking sector
Insurance claims:
The prevalence of fraudulent insurance claims poses a significant challenge for insurers, requiring them to dedicate considerable time and resources to meticulous claim validation. However, traditional methods are often costly and susceptible to vulnerabilities. Fortunately, the emergence of Artificial Intelligence (AI) offers a compelling solution. Leveraging its exceptional pattern recognition abilities, AI can significantly enhance claim processing, enabling:
- Reduced processing time: By automating routine tasks and streamlining workflows, AI can significantly expedite claim resolution, leading to cost savings and improved user experience.
- Enhanced claim settlement accuracy: AI algorithms excel at analyzing vast datasets and identifying anomalies, allowing them to detect fraudulent claims with greater precision. This translates to a more efficient and reliable claims process, safeguarding both insurers and legitimate claimants.
Customer Service and models:
Current customer service models in banking often struggle to meet the demand for timely, personalized, and accurate support. Everyday inquiries can lead to frustrating information gaps, long wait times, and inconsistent service quality. However, the advent of Generative AI presents a transformative opportunity to revolutionize banking customer service. By leveraging AI's capabilities, banks can guarantee seamless access to 24/7 support, regardless of time or location. This empowers customers to resolve issues and access information at their own convenience, significantly improving overall satisfaction and experience. Beyond accessibility, Generative AI unlocks a range of benefits:
- Improved service quality: AI-powered chatbots and virtual assistants can provide accurate and personalized responses to customer inquiries, leading to faster resolution times and enhanced satisfaction.
- Cost-effective scalability: AI solutions can handle large volumes of interactions efficiently, reducing the need for human agents and optimizing operational costs.
- 24/7 availability: AI-powered support never sleeps, ensuring customers receive assistance anytime, anywhere.
Fraud Detection:
AI's exceptional pattern recognition and anomaly detection capabilities enable it to differentiate between legitimate and fraudulent activity with remarkable accuracy. By analyzing vast datasets of financial transactions, AI algorithms can identify suspicious patterns, flag potential threats in real-time, and even automatically initiate countermeasures to block fraudulent activity before it can inflict damage. This proactive approach leads to a multitude of benefits:
- Drastically reduced fraud: AI's ability to pinpoint and prevent fraudulent transactions significantly minimizes financial losses for both banks and their customers.
- Enhanced money laundering detection: AI can uncover complex money laundering schemes by analyzing transaction patterns and identifying unusual financial flows.
- Improved customer service: By swiftly resolving fraudulent incidents and providing real-time fraud alerts, AI fosters enhanced trust and security for customers, leading to a better overall service experience.
- Reduced fraud-related costs: The proactive nature of AI-powered fraud detection minimizes the need for costly investigations and chargebacks, translating to substantial financial savings for banks.
Transaction Monitoring:
Traditional transaction monitoring systems often struggle with a high rate of false positives, frustrating customers and delaying legitimate transactions. However, Artificial Intelligence (AI) offers a promising solution. By analyzing a customer's spending patterns over time and across multiple variables, AI algorithms can accurately identify truly suspicious activity with minimal disruption to genuine transactions. This leads to a two-fold benefit:
- Effective flagging of suspicious activity: AI's superior pattern recognition capabilities significantly reduce false positives, allowing banks to focus resources on genuine threats without hindering legitimate customer activity.
- Improved customer satisfaction: By minimizing unnecessary delays and disruptions, AI-powered transaction monitoring enhances customer satisfaction and fosters trust in the bank's security measures.
Risk profiling:
Mired in resource-draining manual processes, traditional credit risk profiling for loans, cards, and mortgages struggles with speed and accuracy. AI, however, offers a transformative solution. By analyzing vast digital data, AI programs automate risk assessments, leading to:
- Reduced risk: AI's pattern recognition prowess minimizes potential losses.
- Optimized decisions: Data-driven insights enable swift, accurate credit approvals.
- Efficiency gains: Scalable AI handles high volumes, freeing resources and reducing costs.
- Enhanced customer experience: Faster processing and transparency boost satisfaction.
- Embracing AI empowers banks to revamp risk profiling, unlocking benefits for both institutions and customers.
KYC – Customer Due Diligence:
Traditional KYC and Due Diligence, often semi-manual and prone to missed periodic checks, lack real-time risk alerts for potentially problematic customers. AI presents a potent solution. By leveraging comprehensive KYC details and external information, AI can:
- Reduce onboarding friction: Streamlined, AI-powered processes expedite customer onboarding, minimizing friction and improving satisfaction.
- Build a holistic customer profile: AI aggregates data from diverse sources, creating a comprehensive "Customer 360" view for informed decision-making.
- Flag adverse press: Real-time monitoring of external information identifies potential red flags, enhancing risk mitigation.
- Boost accuracy and efficiency: Scalable AI ensures accurate data capture and analysis, leading to faster, more informed decisions.
Regulatory Compliance:
Implementing regulatory changes and comprehensively assessing risk factors in banking has been a time-consuming and manual process, often hampered by data compliance issues. However, Artificial Intelligence (AI) emerges as a powerful solution, empowering compliance analysts to navigate the complex regulatory landscape with greater efficiency and accuracy.
- Faster Implementation: AI algorithms can rapidly analyze vast amounts of regulatory data, identifying relevant changes and automatically suggesting implementation areas. This significantly reduces the time required for manual review and update, ensuring quicker adaptation to evolving regulations.
- Comprehensive Risk Assessment: By analyzing diverse data sources, including customer transactions, market trends, and internal controls, AI can paint a holistic picture of potential risks. This comprehensive risk assessment allows banks to proactively address potential issues before they materialize, mitigating financial losses and reputational damage.
- Data Quality Reconciliation: AI-powered data analysis tools can reconcile data inconsistencies and highlight potential gaps in compliance reporting. This ensures accurate and timely reporting, reducing the risk of regulatory fines and penalties.
Coforge Quasar AI: Reshaping Industries operating in diverse real world scenarios
We assist our clients in a diverse range of Financial and banking sectors, including global banks, marquee central banks, government agencies, fintech companies, cards and payment providers, and global investment managers. 3 decades of experience, 7000 SMEs, the right tech capabilities and partnerships have made this the largest and fastest growing vertical for us. With assets on platform, solutions, and services worth $1.5Tn, 200+ managed platform services, we support 200+ financial institutions, delivering 50K+ complex programs.
The latest addition in this journey is our own platform, Quasar AI, designed to build Enterprise AI capabilities to help our clients in realizing the use cases listed above. The Quasar suite encompasses a range of advanced AI solutions tailored to address diverse data processing and analysis needs. These sophisticated offerings are designed to cater to various aspects of data analytics, utilizing cutting-edge technology to deliver valuable insights. Below, we elaborate on each component of the Quasar suite with their industrial applications.
Quasar Responsible AI
Our proprietary Responsible AI Engine and framework plays a pivotal role in identifying and explaining biases within datasets. Quasar Responsible AI uncovers potential risks and compliance challenges, providing options to govern, mitigate, and remediate third-party risks where necessary. In a world where anti-discrimination and privacy laws are becoming increasingly stringent, our Quasar Responsible AI Platform provides a robust framework for ethical AI integration. Here are some of our Responsible AI solutions:
Our client success stories shaped by Quasar
We empower clients to embrace AI by clarifying their needs and guiding them towards the perfect solution. Our proven approach ensures a product that matches their business goals, closes performance gaps, and leverages data effectively. Here are a few solutions we provided to the clients:
Case study 1:
Problem statement: A pressing issue in the financial sector relates to the rise in anti-money laundering (AML) activities, coinciding with a decline in detection rates. Traditional approaches involve utilizing customer data (KYC details) and behavior patterns for clustering, yet this method encounters limitations. Customers are intricate entities, necessitating ongoing surveillance for unusual behavior, rendering transaction rules inadequate.
Solution: We meticulously analyzed vast amounts of banking transactions, identifying distinct patterns and behaviors. This led to the creation of customer clusters, each akin to a financial fingerprint. Within these clusters, each customer serves as a prototype, representing the typical transaction profile. Suspicion now falls on individuals who fall outside the established norms, either by failing to fit any cluster, exhibiting misalignment within their assigned group, or significantly deviating from the expected prototype behavior. This targeted approach pinpoints potentially fraudulent or unusual activity with unprecedented precision, streamlining investigations and safeguarding the financial security.
Result: This in-depth examination of customer behavior yields several benefits, including enhanced fraud management, improved fraud detection rates, a noteworthy reduction in false positives, more efficient workload management, and a decrease in manual intervention. This approach equips financial institutions with a more robust and adaptive framework for combatting AML activities effectively.
Case study 2:
Problem statement: A prominent global bank encountered a significant obstacle in its risk management process. The bank relied on annual supplier-issued reports, validated by external auditors, to define and assess supplier controls. These reports were crucial for their supplier risk management framework. However, the manual analysis of these reports, comparing them year-over year to identify control changes, proved cumbersome and error-prone, hindering efficient risk assessment. To address this challenge, the bank sought a more streamlined solution.
Solution: We leveraged machine learning techniques to efficiently compare the reports and identify changes. The process included sentence extraction and preprocessing removing irrelevant characters, applying text normalization techniques, and tokenization. An LLM model was employed to measure the semantic similarity between sentences from corresponding reports. Sentences that match completely are removed and non-matching sentences are highlighted in the source file. A summary report is generated, listing all non-matching sentences and their locations within the respective PDFs. This report provides a concise overview of potential control modifications.
Result: This ML solution presents a transformative paradigm shift, empowering a faster, more accurate, and transparent risk assessment process. Automated processes seamlessly handle report comparison, dramatically reducing time and effort invested, leading to enhanced efficiency and high accuracy.
Case study 3:
Problem statement: A leading National Commercial bank faced a challenge to develop a robust system capable of accurately extracting relevant information from cheques, ensuring seamless integration into financial processes. This involves addressing issues related to handwriting recognition, varied formats, and potential errors, with the goal of enhancing efficiency and accuracy in financial transactions.
Solution: Our innovative approach to tackling the extraction of crucial information from cheques involved leveraging Yolo for signature extraction. Yolo, known for its real-time object detection capabilities, provides a robust foundation for identifying and isolating signatures on cheques efficiently. Building on this, we implemented a trained spacy model to carry out the extraction of key details such as the payee’s name, serial number, account number, amount, and accuracy. The Spacey model, honed through meticulous training, demonstrated remarkable accuracy in discerning and isolating specific elements within the cheque. This dual-model strategy combined the strength of Yolo for signature identification with the nuanced understanding of Spacey for extracting textual information. The synergy between these models ensured a comprehensive retrieval of relevant data from the diverse formats that cheques may present.
Result: Our system not only streamlines the extraction process but also enhances accuracy minimizing errors in crucial financial data. By integrating YOLO and Spacey, we have developed sophisticated solution that stands at the forefront of technology, addressing the intricacies of cheque processing for improved efficiency in financial workflows.
Challenges and Limitations
- Security and Privacy: The vast financial data entrusted to banks necessitates robust security measures for AI systems. These systems must be impenetrable against cyberattacks and data breaches, safeguards critical in an increasingly digital landscape. However, ensuring data privacy and compliance with regulations like GDPR presents significant challenges in the context of AI. As these systems learn and adapt based on data, striking a balance between leveraging their analytical power and adhering to strict data protection regulations becomes crucial. This requires careful consideration of data anonymization and encryption, differential privacy, transparency and explainability, and continuous monitoring and auditing.
- Misinformation and bias amplification: AI algorithms trained on biased data can inadvertently perpetuate and even amplify existing societal inequalities, leading to inaccurate risk assessments and potentially unfair lending practices. This challenge necessitates a proactive approach to mitigate bias at multiple levels requiring a commitment to ethical development, responsible data governance, and continuous improvement. By actively mitigating bias, we can ensure that AI empowers fair and equitable financial practices for all.
- Regulatory environment and social acceptance: The evolving landscape of regulations surrounding data privacy, algorithmic bias, and ethical considerations can pose significant challenges. Organizations must stay abreast of these regulations and ensure their AI solutions comply with all relevant legal requirements. Public perception and trust in AI technologies also play a crucial role in their adoption. Concerns about job displacement, privacy infringements, and potential misuse of AI can hinder widespread acceptance. Addressing these concerns through transparent communication, responsible development practices, and ethical application of AI is crucial.
- Impact assessment: A rigorous and continuous evaluation of both the effectiveness and return on investment (ROI) associated with such initiatives is important. These assessments serve as critical checkpoints for ensuring judicious allocation of resources. By regularly measuring the value generated by advanced analytics solutions, organizations can optimize their resource allocation, directing investments towards initiatives with demonstrated efficacy and profitability.
The Way Forward
AI is rapidly reshaping the landscape of banking and finance and will continue to do so. Banks and financial institutions are leveraging its power to streamline operations through automation, personalize customer interactions with intelligent solutions, and drive growth through innovative data-driven insights. Several leading banks such as JPMorgan Chase, Mastercard, Bank of America have used AI and ML techniques for processes like automated contract reviews, identifying fraudulent transactions, and providing personalized recommendations.
Few of the potential use cases for banking are:
- Banking of Things:
The exponential rise in internet-connected devices ushers in the era of "Banking of Things" (BoT), a data-driven infrastructure that transforms everyday objects into financial touchpoints. By leveraging edge computing, BoT can harness the vast data streams generated by these devices to offer personalized services and make informed financial decisions. For example, a smart fridge, empowered by BoT, automatically sensing depleting milk supplies and triggering a purchase order via an online store, seamlessly integrated with its embedded digital wallet or linked bank account. This example exemplifies the transformative potential of BoT, weaving data into the fabric of everyday life and enabling frictionless, context-aware financial transactions. - Optimizing loan management
Leveraging the power of data, AI can revolutionize loan approval by automating decision-making based on factors like age, income, expenditure, average balance, and debt levels. This data-driven approach can streamline the process, significantly reducing time and resource requirements for both banks and borrowers. By analyzing a comprehensive financial profile, AI models can identify the most suitable loan options while mitigating risk for both parties. This translates into quicker approvals, increased efficiency, and a more risk-adjusted lending landscape. - Reduced missing payments
Digital banking apps, empowered by AI, can revolutionize customer financial well-being through proactive payment reminders and personalized financial management tools. By leveraging transaction data and spending habits, AI can predict potential shortfalls and strategically notify customers of upcoming bills and car insurance payments. This early intervention empowers customers to avoid missed payments, fostering a sense of financial security and control. This proactive approach not only minimizes the risk of default but also elevates customer experience, potentially boosting loyalty and Net Promoter Scores (NPS). The "Customer Alert tool (forecasting spend)" exemplifies this paradigm shift, empowering customers to make informed financial decisions and navigate their financial landscape with confidence. - Bolstering Transaction Security
Leveraging the analytical prowess of AI, banks can proactively safeguard user transactions against fraudulent activity and protect their financial well-being. By analyzing consumption patterns and cross-referencing data from various sources, AI can identify anomalous transactions in real-time. For instance, if a bank card linked to a specific geographical location exhibits activity in another city, AI can trigger an alert, notify the user, and request verification. This swift intervention not only reduces identity theft but also acts as a powerful deterrent against sophisticated financial crimes like money laundering. This "Point of transaction assessment tool" represents a paradigm shift in financial security, empowering banks to significantly reduce fraud and money laundering incidents, ultimately fostering a safer and more secure financial ecosystem for all. - Virtual Wealth Management advisor
The virtual advisor can empower customers to make informed decisions, fostering financial literacy and a sense of control over their future. It's a constant companion, a tireless analyst, all rolled into one. With this AI co-pilot with the customer, navigating the path towards financial well-being becomes a more confident, informed, and ultimately, more rewarding journey.
By actively embracing AI and continuously exploring its potential across diverse applications, banks and financial institutions can confidently navigate the evolving landscape and solidify their competitive edge. Coforge, recognized for its unwavering commitment to client success, consistently strives to be a trusted partner in this transformative journey, providing the expertise and resources necessary for the clients to harness the full potential of AI and achieve sustainable growth.
References
https://www.coforge.com/industries/banking-and-financial-services
Take the Next Step with Quasar AI!
Ready to unlock the power of AI in your banking operations? Here’s how:
Explore Quasar AI: Dive deeper into the capabilities of our cutting-edge AI platform. Visit our website to learn about its features, case studies, and success stories.
Request a Demo: Curious to see Quasar AI in action? Contact our team to schedule a personalized demo.
Connect with Us: Have questions or need more information? Reach out! We’re here to assist you. Email us at xyz@coforge.com.
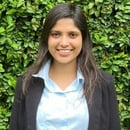
Shalu Chaudhary, AI Program Manager at Coforge, is passionate about Advanced Analytics and GenAI and wishes to explore their full potential in the fields of banking, finance and insurance
Related reads.
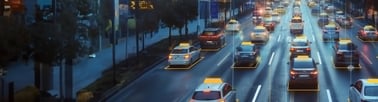
About Coforge.
We are a global digital services and solutions provider, who leverage emerging technologies and deep domain expertise to deliver real-world business impact for our clients. A focus on very select industries, a detailed understanding of the underlying processes of those industries, and partnerships with leading platforms provide us with a distinct perspective. We lead with our product engineering approach and leverage Cloud, Data, Integration, and Automation technologies to transform client businesses into intelligent, high-growth enterprises. Our proprietary platforms power critical business processes across our core verticals. We are located in 23 countries with 30 delivery centers across nine countries.