Abstract
Premium is often considered to be the prime cause of customer attrition in the insurance sector. The traditional rule-based engine in actuarial science majorly relies on the past claims and expense data to compute the premium. This baseline price can be used as an input for the price optimization model to predict the optimal quote price within the underwriter’s marginal constraints. Here Coforge presents a more holistic solution with descriptive and diagnostically active dashboards for deeper insights. A dynamic interface augmented with predictive and prescriptive abilities presents various what-if scenarios to make more informed decisions in business.
Persistent Need for Optimal Pricing Strategy
Insurers need to lay down several risk assessment and risk management strategies to reduce even marginal errors that can yield huge losses to them. Actuarial science is thus building solutions to bring high precision in their operations. One of the key aspects for them is to perform careful due diligence for their product’s pricing. Under-pricing can reduce their expected profits and overpricing can raise customer churn rate, thus impacting their overall sales. Keeping this in mind, multiple types of research have been conducted to provide key factors that have been extensively used to build a traditional rule-based engine for computing the point estimate of their product’s price (Sames, 2006) (Bastien). However, the possibility of analyzing the distribution of price range within the underwriter margins remain unexplored. Secondly, price sensitivity is multi-dimensional comprising various internal and external factors along with strict government regulations and compliances (especially in US and UK markets) which makes it even more difficult to assess. Thus till now, traditional actuarial pricing is only cost-driven as it majorly employs historical claims and expenses data to estimate future risks and profits. However, there is other relevant information such as; customer behavior patterns, demographics, agent’s performance, competitor outlook which could majorly impact the attrition rates. Thus, a more comprehensive view could be presented by considering these factors to get deeper insights and more informed decisions.
Modeling Demand
• Traditional actuarial pricing gives only a rule-based point estimate of the premium.
• Distribution of possible price ranges needs to be analyzed based on customer needs or segmentation.
• Current modeling approaches mostly rely only on the historical claim data and expenses.
• Major dimensions of data remain unmapped such as customer experiences, agent's performance, etc.
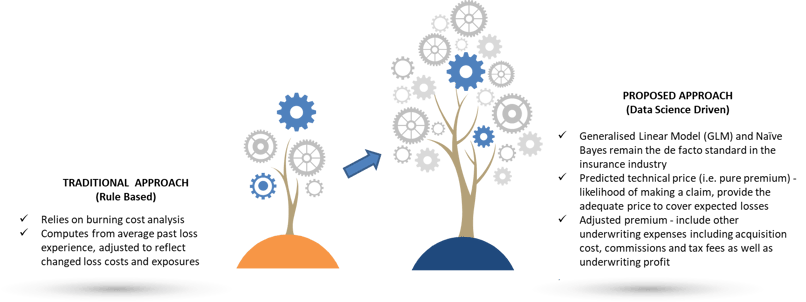
Figure 1: Traditional versus Proposed Approach
Price Optimizer: An Explainable AI Solution
Price optimization offers an optimally modified premium suited to each customer or segment based on myriad of data points in order to maximize customer retention or/and insurer’s profitability while considering real time constraints. This process initiates from traditionally computed underwriter’s premium base price and then varies within underwriter’s set margins. Data science engine predicts the best quote premium with set objectives and constraints for each policy. This allows the end users to understand the price elasticity and customize it to the level of individual policy.
Model Operationalization
The current solution is built for the group life insurance for two product lines - basic life and long-term disability. Data points from operations, sales, marketing, underwriters are integrated to get past customer experience & demographics, agency information, claim experience, loss, expenses, profits, premium and product related information. A churn model for customer retention and conversion model for customer acquisition was built using Generalized Linear Models (GLM) and Naïve Bayes algorithms which are de-facto standard in insurance industry (Mark Goldburd, 2016) (Karl P Murphy, 2000). GLM can be used for prediction and classification to estimate regression models for various distributions like exponential, Gaussian, poisson, binomial, and gamma with various link functions (Tomas Nykodym, 2019) (Giorgio Alfredo Spedicato, 2018). These models are then supplemented with the price optimization model to assess the price tolerance at an individual policy level. This competitive modelling sets up the price, which maximizes the profit from retained customers and optimal discount is computed to prevent the churn of customers. Various business related what-if scenarios were designed to demonstrate the capability of the solution.
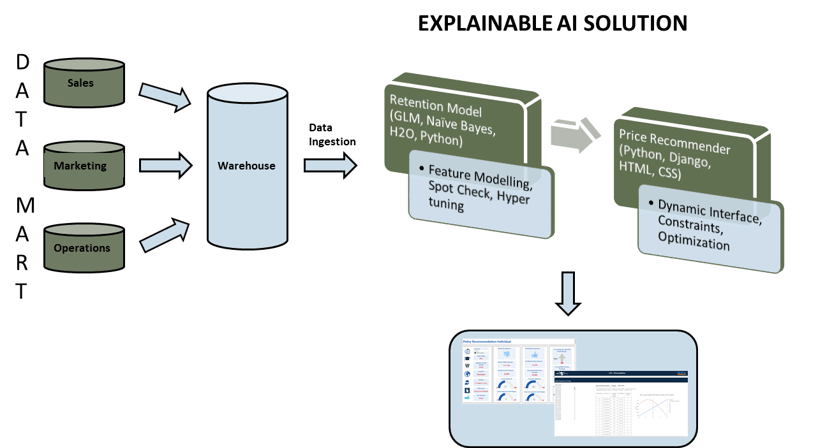
Figure 2: Operationalization of the Solution
Key Benefits and Features
Our explainable AI solution offers dynamic interface for the insurers to tweak crucial risk and coverage parameters and get the new quote with maximal profits and reduced churn. This offering provides active dashboards for portfolio insights and diversity, agency information, policy level information, customer and claim experience and quote recommendation engine. Dynamic interface allows the user to assess various what-if scenarios.
• Business Intelligence Reporting with Predictive and Prescriptive Dynamic Interface
• Easy Portfolio Analysis and Management
• Identification of profitable and loss-making segments in single click
• Policy level highlights to investigate and strategize their retention with profits
• Customized risk mitigation or Personalized policy designing
• Linking attrition with customer experience and agent/agency performance
• Easy integration with customer data marts
• Easily deployable solution on private/public cloud platforms
• Economic prices compared to other available solutions
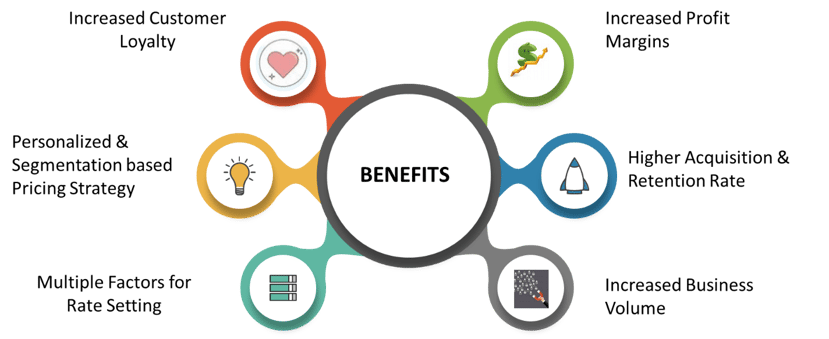
Figure 3: Potential Benefits of Proposed Price Optimization Solution to the Business
Conclusion
In a scenario of cutting-edge competition, harmonizing product risk with best price quote, customized at the level of each policy presents new opportunities to business to stay profitable in the long run using machine learning. Not only this, given the capability to change the risk, coverages and benefits related parameters best suited to each of the target customer and get the real time updated optimal quote against the same with no time is the unique selling point of this solution. Lot of retention related strategies can be laid down from the agency level, customer experience level, segment level, and policy level and portfolio level dashboards. In future, other attrition related parameters could also be explored along with the up-selling & cross-selling opportunities.
References
- Bastien, M. The Pricing of Group Life Insurance Schemes. Actuariesindia.
- Giorgio Alfredo Spedicato, C. D. (2018). Machine Learning Methods to Perform Pricing Optimization. A Comparison with Standard GLMs. Society of Actuaries.
- Karl P Murphy, M. J. (2000). Using Generalized Linear Models to Build Dynamic Pricing Systems for Personal Lines Insurance . Casualty Actuarial Society.
- Mark Goldburd, A. K. (2016). Generalized linear models for insurance rating. Casualty Actuarial Society .
- Sames, S. R. (2006). Group Life Insurance Mortality and Morbidity Study . Society of Actuaries.
- Tomas Nykodym, T. K. (2019, December). https://www.h2o.ai/resources/. Retrieved from www.h2o.ai: http://docs.h2o.ai/h2o/latest-stable/h2o-docs/booklets/GLMBooklet.pdf