Kafka is a community distributed, event-driven, streaming platform and was originally created and open sourced by LinkedIn in 2011. Kafka is based on an abstraction of a distributed commit log.
What are the 3 key capabilities of Kafka as a streaming platform?
- Publish and subscribe to streams of records.
- Store streams of records in a fault-tolerant durable way.
- Process streams of records as they occur.
What kind of applications can you build with Kafka?
- Real-time streaming data pipelines that reliably ingest and publish data between systems or applications.
- Real-time streaming applications that transform or react to the streams of data.
Which companies are using Kafka?
- 60% of S&P 500 companies.
- LinkedIn, Uber, Spotify, Netflix, Airbnb, Twitter, Slack, Pinterest, Yahoo etc.
- Widely used by most companies in banking, retail, ecommerce etc.
How Does Kafka Work? Kafka’s Key Concepts
- Kafka is as a distributed platform, runs as a cluster on one or more servers that can span multiple datacentres.
- The Kafka cluster stores streams of records in categories called topics. A topic is a stream of records; it is a category or feed name to which records are published.
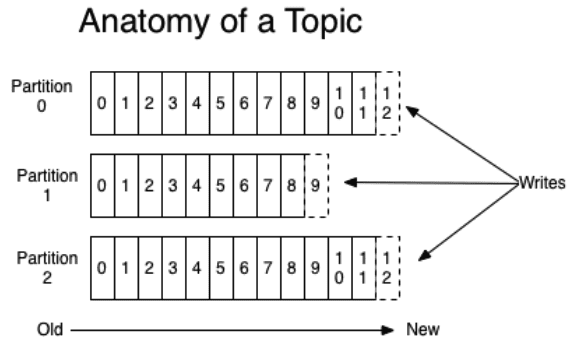
- Each record in the stream consists of a key, a value, and a timestamp
- For each topic, Kafka maintains a partitioned log.
Each partitioned log is an ordered, immutable sequence of records that is continually appended to—a structured commit log. The records in the partitions are each assigned a sequential id number called the offset that uniquely identifies each record within the partition.
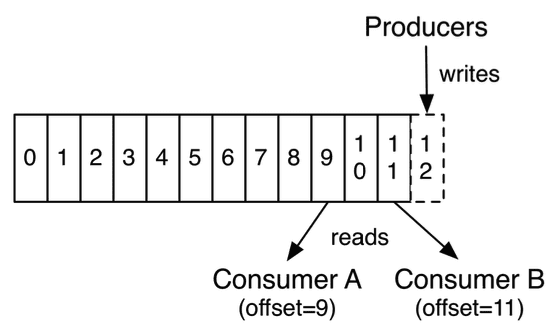
What are the Main Five Kafka Components?
- The Producer API allows an application to publish a stream of records to one or more Kafka topics.
- The Consumer API allows an application to subscribe to one or more topics and process the stream of records produced to them.
- The Streams API allows an application to act as a stream processor, consuming an input stream from one or more topics and producing an output stream to one or more output topics, effectively transforming the input streams to output streams.
- The Connector API allows building and running reusable producers or consumers that connect Kafka topics to existing applications or data systems. For example, a connector to a relational database might capture every change to a table.
- The Admin API allows managing and inspecting topics, brokers and other Kafka objects.
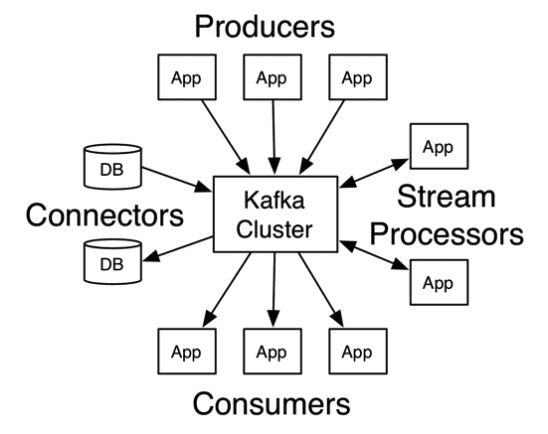
How is Kafka different from a Messaging System?
How does the Kafka streaming platform compare to a traditional enterprise messaging system?
Messaging traditionally has two models: queuing and publish-subscribe. In a queue, a pool of consumers may read from a server and each record goes to one of them; in publish-subscribe the record is broadcast to all consumers. Each of these two models has a strength and a weakness. The strength of queuing is that it allows you to divide up the processing of data over multiple consumer instances, which lets you scale your processing. Unfortunately, queues aren't multi-subscriber—once one process reads the data it's gone. Publish-subscribe allows you broadcast data to multiple processes, but has no way of scaling processing since every message goes to every subscriber.
The consumer group concept in Kafka generalises these two concepts. As with a queue the consumer group allows you to divide up processing over a collection of processes (the members of the consumer group). As with publish-subscribe, Kafka allows you to broadcast messages to multiple consumer groups.
The advantage of Kafka's model is that every topic has both these properties—it can scale processing and is also multi-subscriber—there is no need to choose one or the other.
Kafka has stronger ordering guarantees than a traditional messaging system, too.
A traditional queue retains records in-order on the server, and if multiple consumers consume from the queue then the server hands out records in the order they are stored. However, although the server hands out records in order, the records are delivered asynchronously to consumers, so they may arrive out of order on different consumers. This effectively means the ordering of the records is lost in the presence of parallel consumption. Messaging systems often work around this by having a notion of "exclusive consumer" that allows only one process to consume from a queue, but of course this means that there is no parallelism in processing.
Kafka does it better. By having a notion of parallelism—the partition—within the topics, Kafka is able to provide both ordering guarantees and load balancing over a pool of consumer processes. This is achieved by assigning the partitions in the topic to the consumers in the consumer group so that each partition is consumed by exactly one consumer in the group. By doing this we ensure that the consumer is the only reader of that partition and consumes the data in order. Since there are many partitions this still balances the load over many consumer instances. Note however that there cannot be more consumer instances in a consumer group than partitions.
Kafka for Streams Storage
Any message queue that allows publishing messages decoupled from consuming them is effectively acting as a storage system for the in-flight messages. What is different about Kafka is that it is a very good storage system.
Data written to Kafka is written to disk and replicated for fault-tolerance. Kafka allows producers to wait on acknowledgement so that a write isn't considered complete until it is fully replicated and guaranteed to persist even if the server written to fails.
The disk structures Kafka uses scale well—Kafka will perform the same whether you have 50 KB or 50 TB of persistent data on the server.
As a result of taking storage seriously and allowing the clients to control their read position, you can think of Kafka as a kind of special purpose distributed filesystem dedicated to high-performance, low-latency commit log storage, replication, and propagation.
Kafka for Stream Processing
It isn't enough to just read, write, and store streams of data, the purpose is to enable real-time processing of streams.
In Kafka a stream processor is anything that takes continual streams of data from input topics, performs some processing on this input, and produces continual streams of data to output topics.
For example, a retail application might take in input streams of sales and shipments, and output a stream of reorders and price adjustments computed off this data.
It is possible to do simple processing directly using the producer and consumer APIs. However for more complex transformations Kafka provides a fully Streams API. This allows building applications that do non-trivial processing that compute aggregations off of streams or join streams together.
This facility helps solve the hard problems this type of application faces: handling out-of-order data, reprocessing input as code changes, performing stateful computations, etc.
The streams API builds on the core primitives Kafka provides: it uses the producer and consumer APIs for input, uses Kafka for stateful storage, and uses the same group mechanism for fault tolerance among the stream processor instances.
Putting All the Pieces Together
This combination of messaging, storage, and stream processing may seem unusual but it is essential to Kafka's role as a streaming platform.
A distributed file system like HDFS allows storing static files for batch processing. Effectively a system like this allows storing and processing historical data from the past.
A traditional enterprise messaging system allows processing future messages that will arrive after you subscribe. Applications built in this way process future data as it arrives.
Kafka combines both of these capabilities, and the combination is critical both for Kafka usage as a platform for streaming applications as well as for streaming data pipelines.
By combining storage and low-latency subscriptions, streaming applications can treat both past and future data the same way. That is a single application can process historical, stored data but rather than ending when it reaches the last record it can keep processing as future data arrives. This is a generalized notion of stream processing that subsumes batch processing as well as message-driven applications.
Likewise for streaming data pipelines the combination of subscription to real-time events make it possible to use Kafka for very low-latency pipelines; but the ability to store data reliably make it possible to use it for critical data where the delivery of data must be guaranteed or for integration with offline systems that load data only periodically or may go down for extended periods of time for maintenance. The stream processing facilities make it possible to transform data as it arrives.
Note: To give you the most accurate and up-to-date description of Kafka, we considered two of the most trusted resources: Confluent and The Apache Software Foundation.
Other useful links:
Journey to the event-driven business with Kafka
Coforge Expert Kafka Services
Coforge Confluent Services
Related reads.
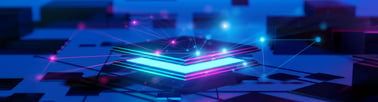
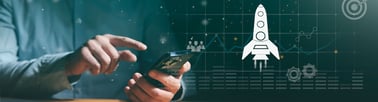
About Coforge.
We are a global digital services and solutions provider, who leverage emerging technologies and deep domain expertise to deliver real-world business impact for our clients. A focus on very select industries, a detailed understanding of the underlying processes of those industries, and partnerships with leading platforms provide us with a distinct perspective. We lead with our product engineering approach and leverage Cloud, Data, Integration, and Automation technologies to transform client businesses into intelligent, high-growth enterprises. Our proprietary platforms power critical business processes across our core verticals. We are located in 23 countries with 30 delivery centers across nine countries.